Revolutionizing Bilingual Text Embeddings with Multi-Task Contrastive Learning
Our new paper explores how our Spanish-English and German-English models use multi-task contrastive learning and a sophisticated data pipeline to master language understanding and cross-lingual efficiency for texts up to 8192 tokens
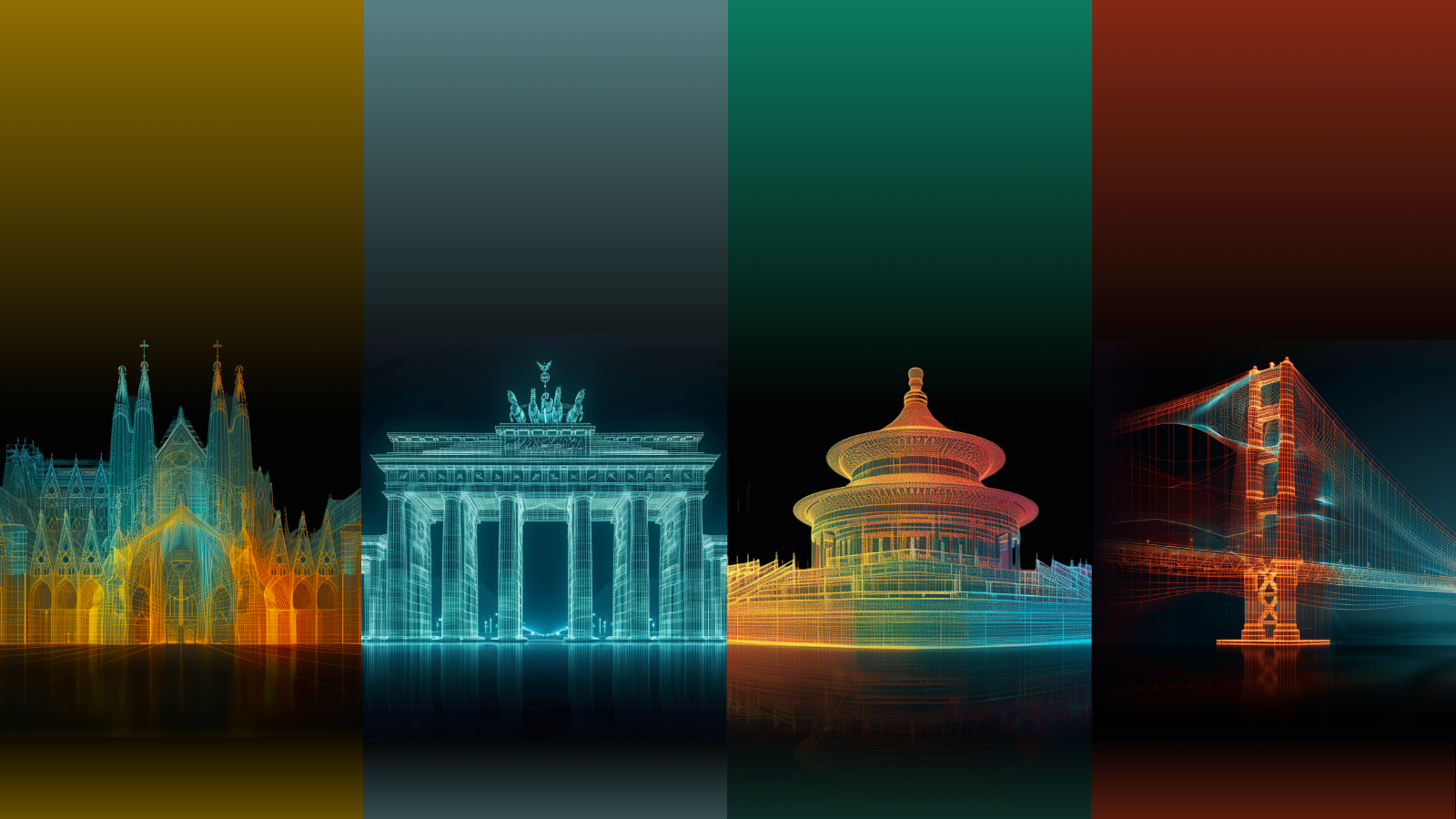
In our recent paper, Multi-Task Contrastive Learning for 8192-Token Bilingual Text Embeddings, we detailed our development of German-English and Spanish-English bilingual text embedding models.
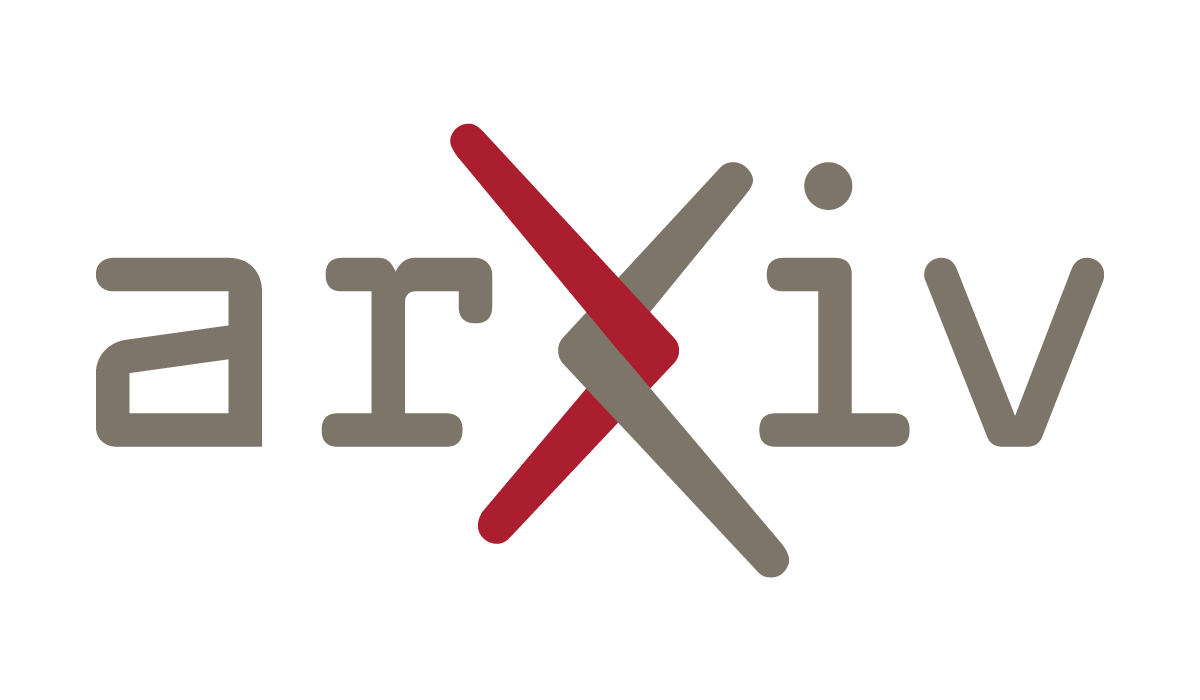
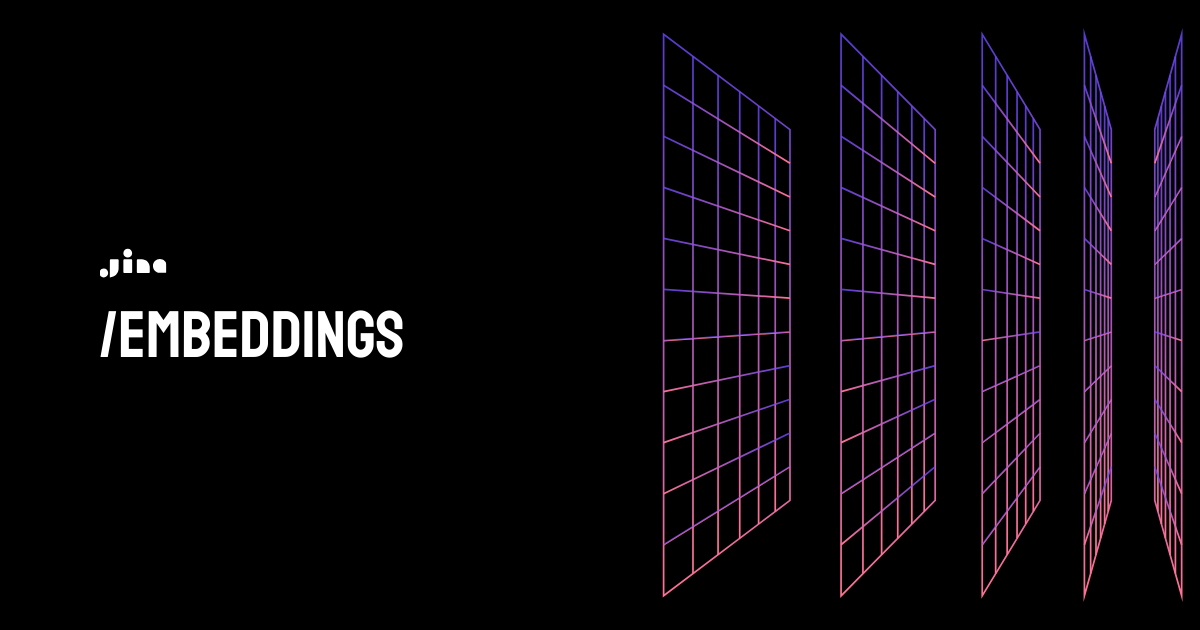
Our approach utilizes multi-task contrastive learning and advanced data curation pipeline, focusing on bilingual capabilities while extending to support 8192 tokens in length. This method allows our models to excel in understanding target languages and in conducting cross-lingual evaluations efficiently.
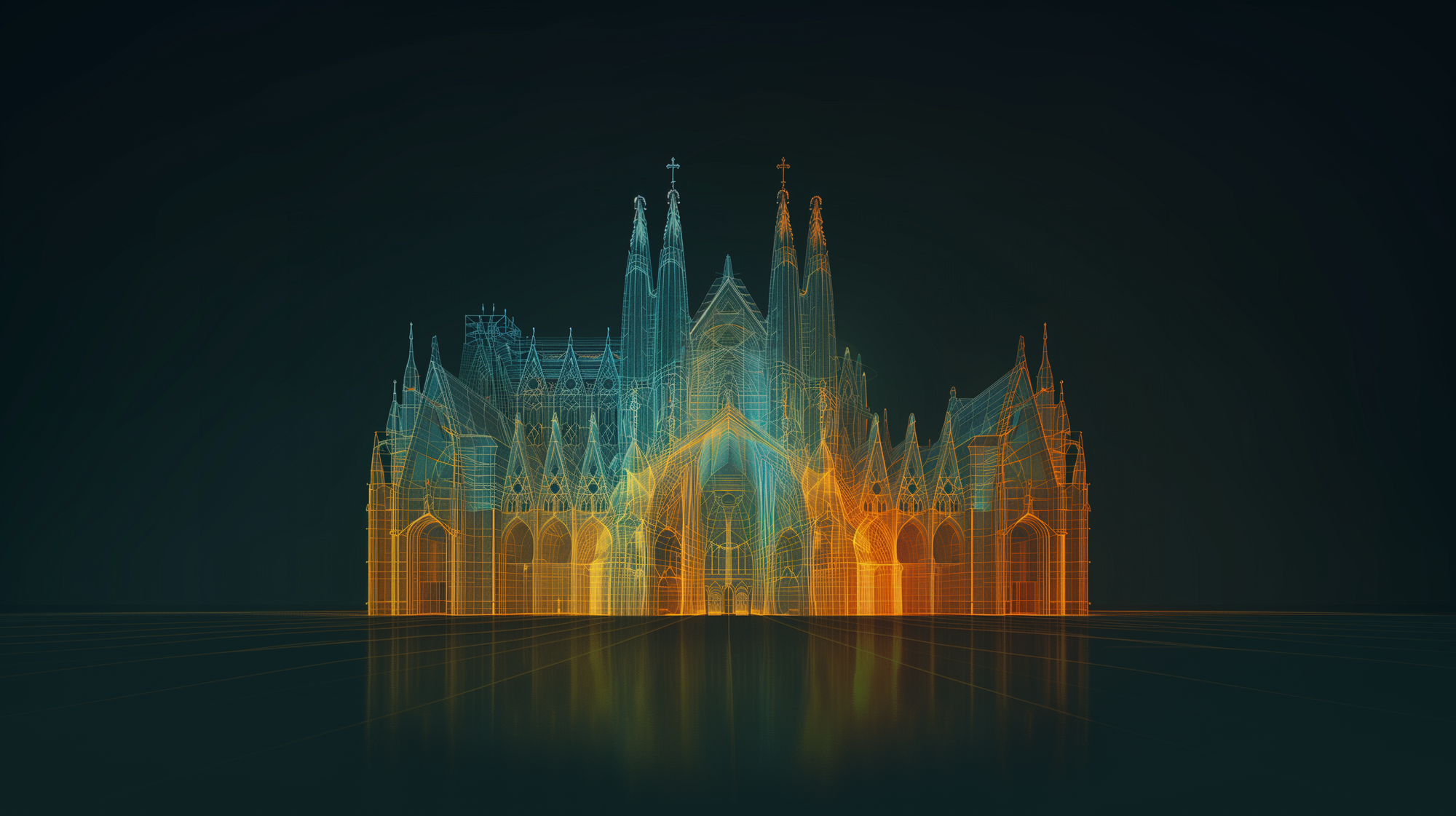
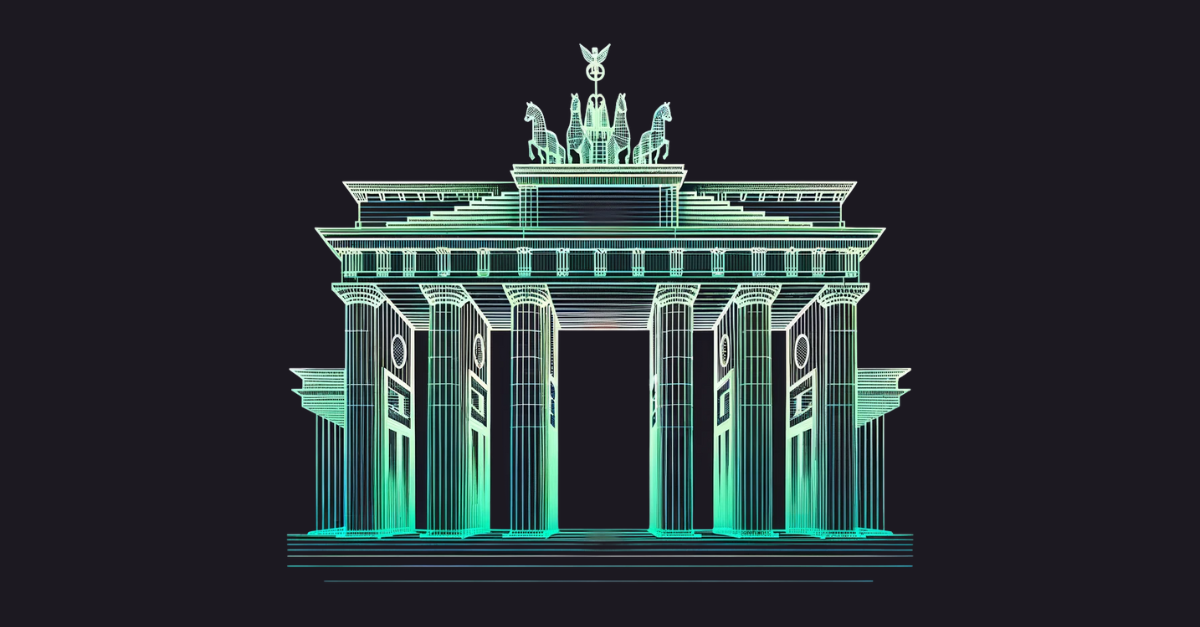
In addition to the bilingual models covered in the paper, we have also developed bilingual Chinese-English and monolingual English models. These additions showcase our commitment to covering a broad spectrum of linguistic needs and furthering our capabilities in language processing.
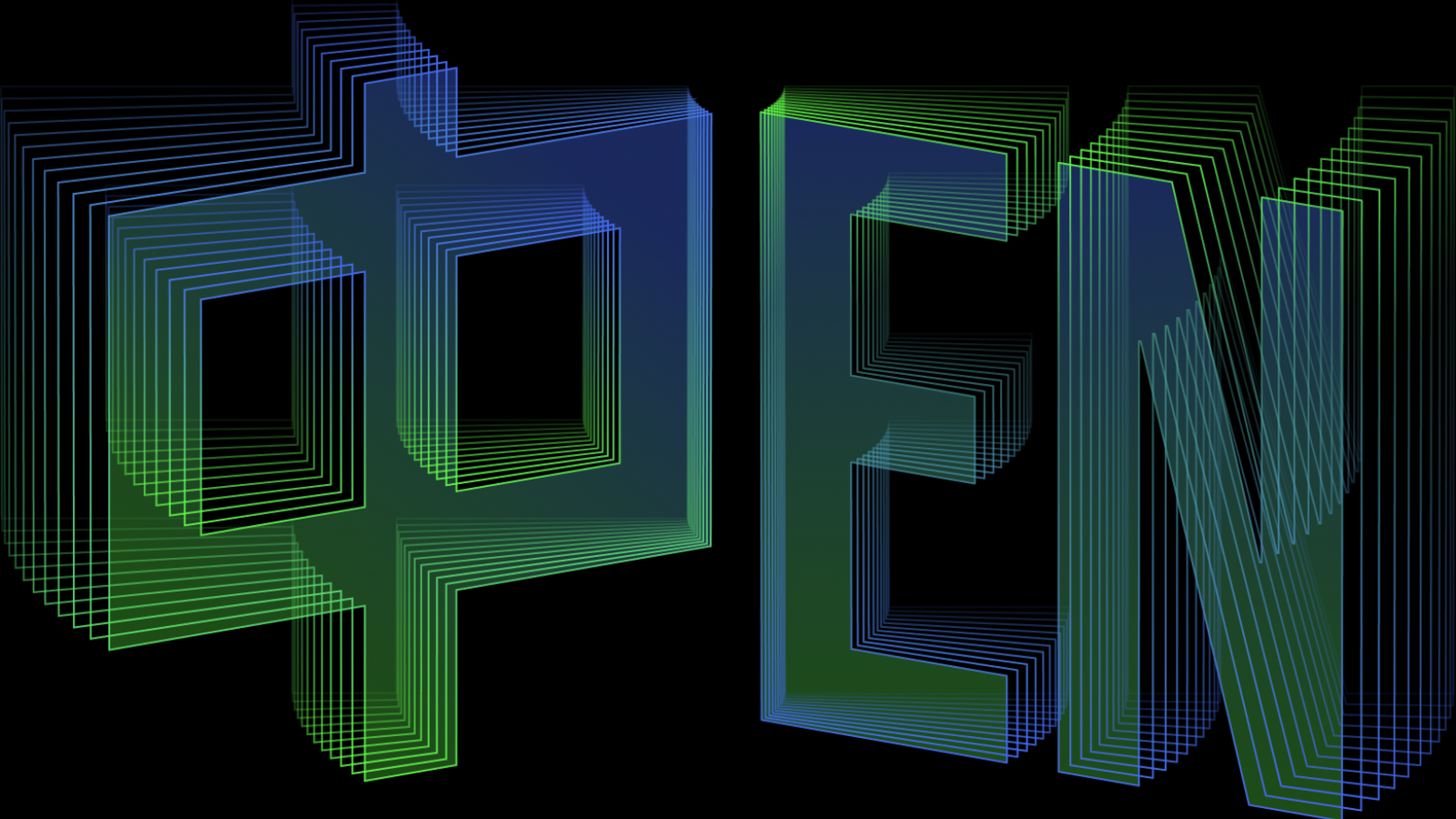
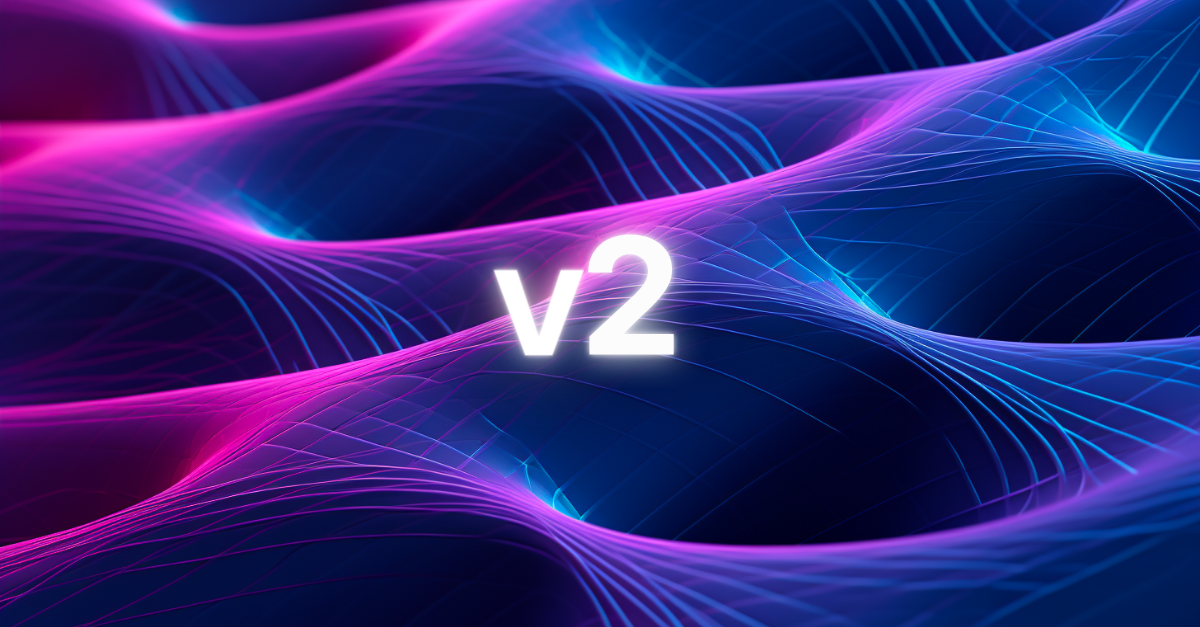
Our bilingual models are characterized by their efficiency, operating with optimized vocabulary sizes to require fewer parameters and less memory. This efficiency underscores our dedication to creating powerful yet resource-efficient tools for language processing.
Following the release of our paper, we expanded the Massive Text Embedding Benchmark (MTEB) to include benchmarks for our English-German and English-Spanish embedding models. This expansion is part of our effort to stimulate further research and advancements in text embedding technologies for non-English languages.
At Jina AI, our aim is to enhance the processing and understanding of multiple languages, contributing to the NLP field with our developments in bilingual and monolingual text embedding models.