The 1950-2024 Text Embeddings Evolution Poster
Take part in celebrating the achievements of text embeddings and carry a piece of its legacy with you.
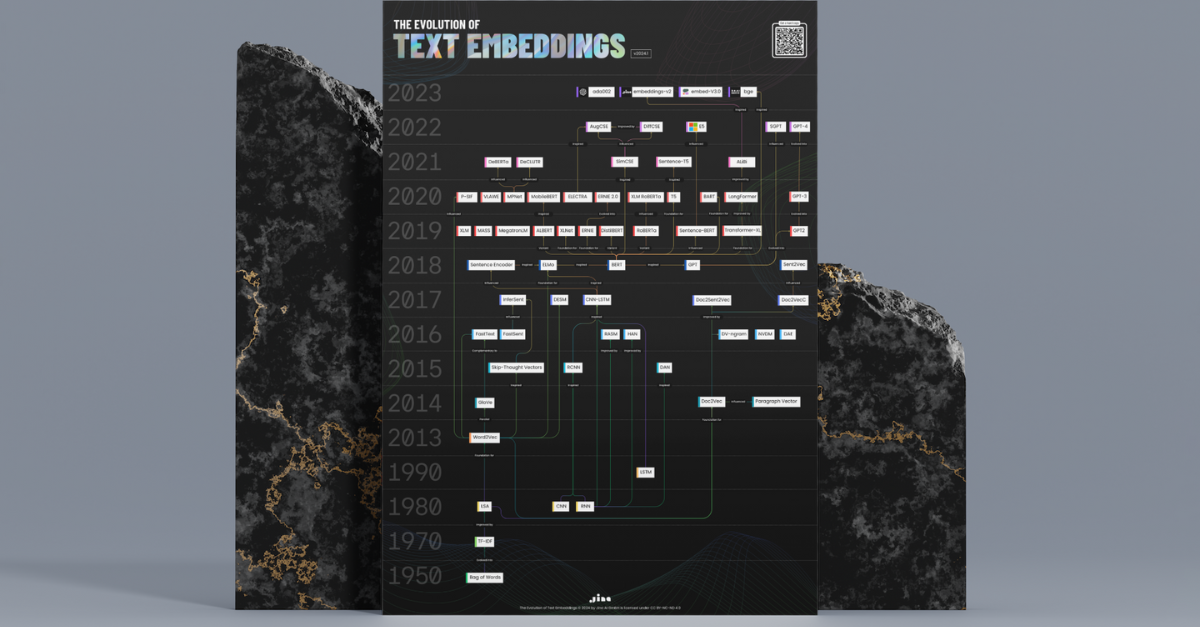
Embark on a journey through time with our latest infographic poster, showcasing the remarkable evolution of text embeddings from 1950 to 2024. This visually striking piece is not just a testament to technological progress; it's a guide that traces the lineage of innovations that have revolutionized how we represent text data.
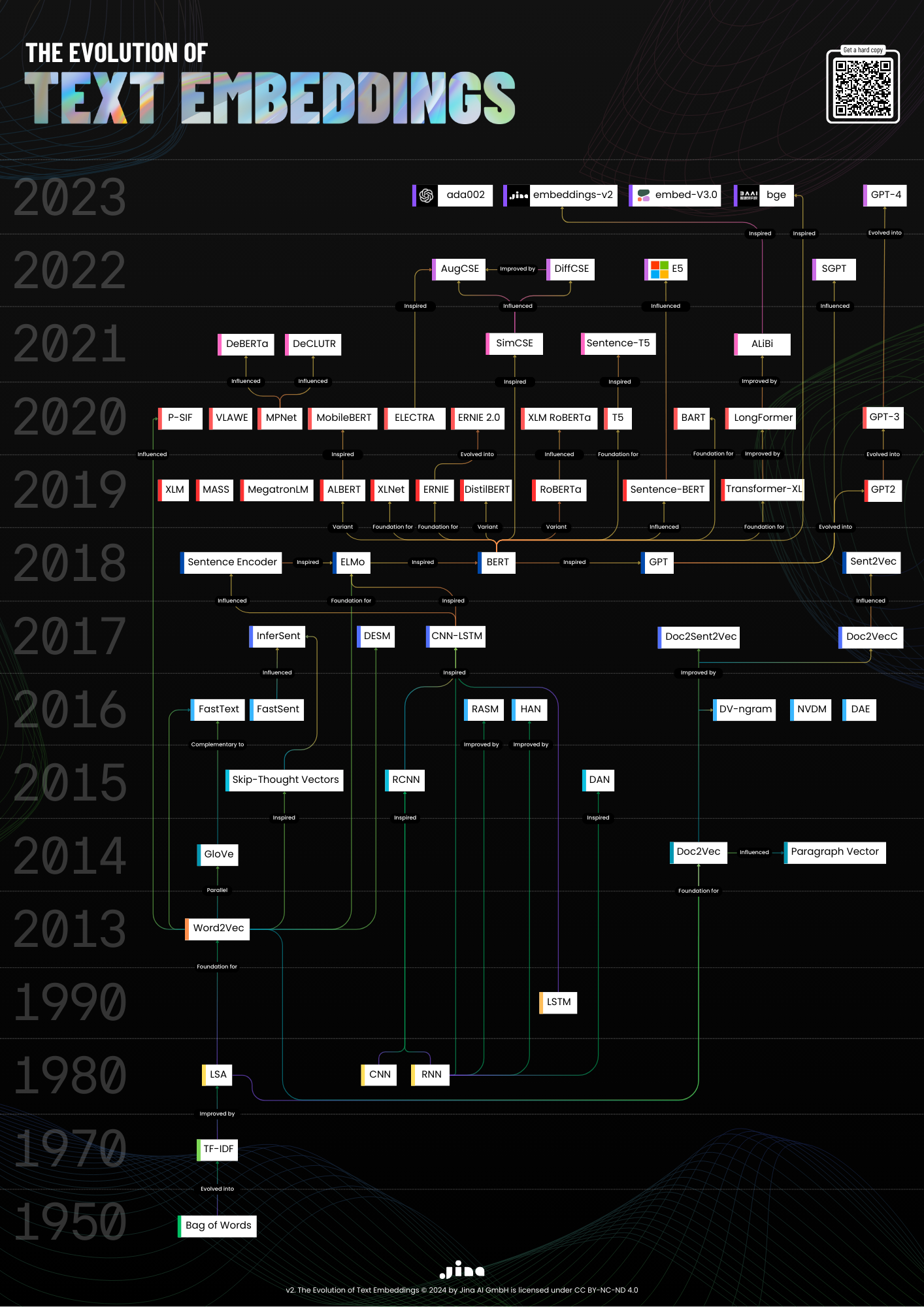
A Chronicle of Innovation
From the foundational Bag of Words model to the 8K token-length jina-embeddings-v2 and everything in between, our poster captures each step in advancing text embeddings. Designed with precision, it highlights how each breakthrough built upon its predecessors, leading us to today's embeddings-driven applications.
Designed for Enthusiasts and Professionals Alike
Whether you're a data scientist, a software engineer, an AI researcher, or simply a technology enthusiast, this poster is a must-have. It's more than just a visual treat; it's an educational tool that breaks down complex developments into an accessible format.
Bring Home a Piece of AI History
For those who appreciate the tactile feel of quality, you can own this piece of history by purchasing a hard copy. Imagine this stunning poster adorning your living room wall, sparking conversations and inspiring future innovators.
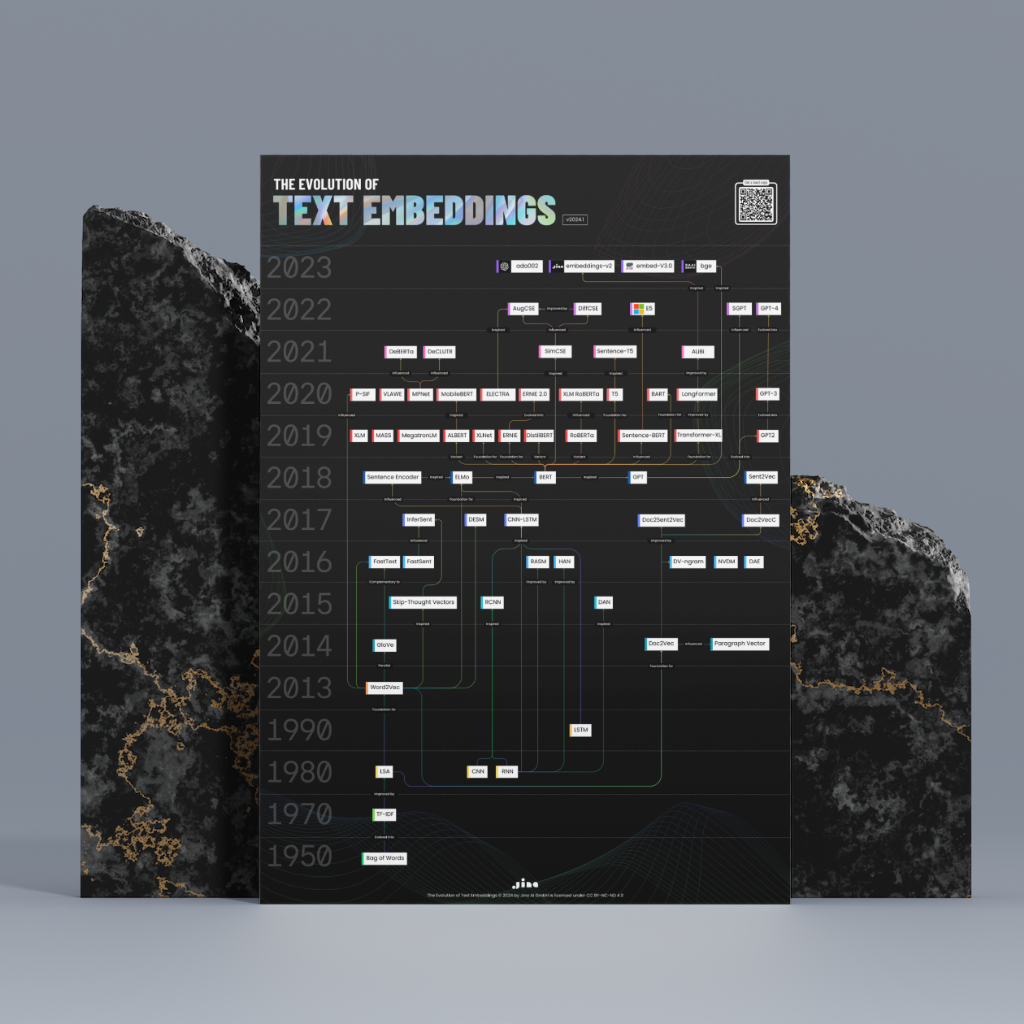
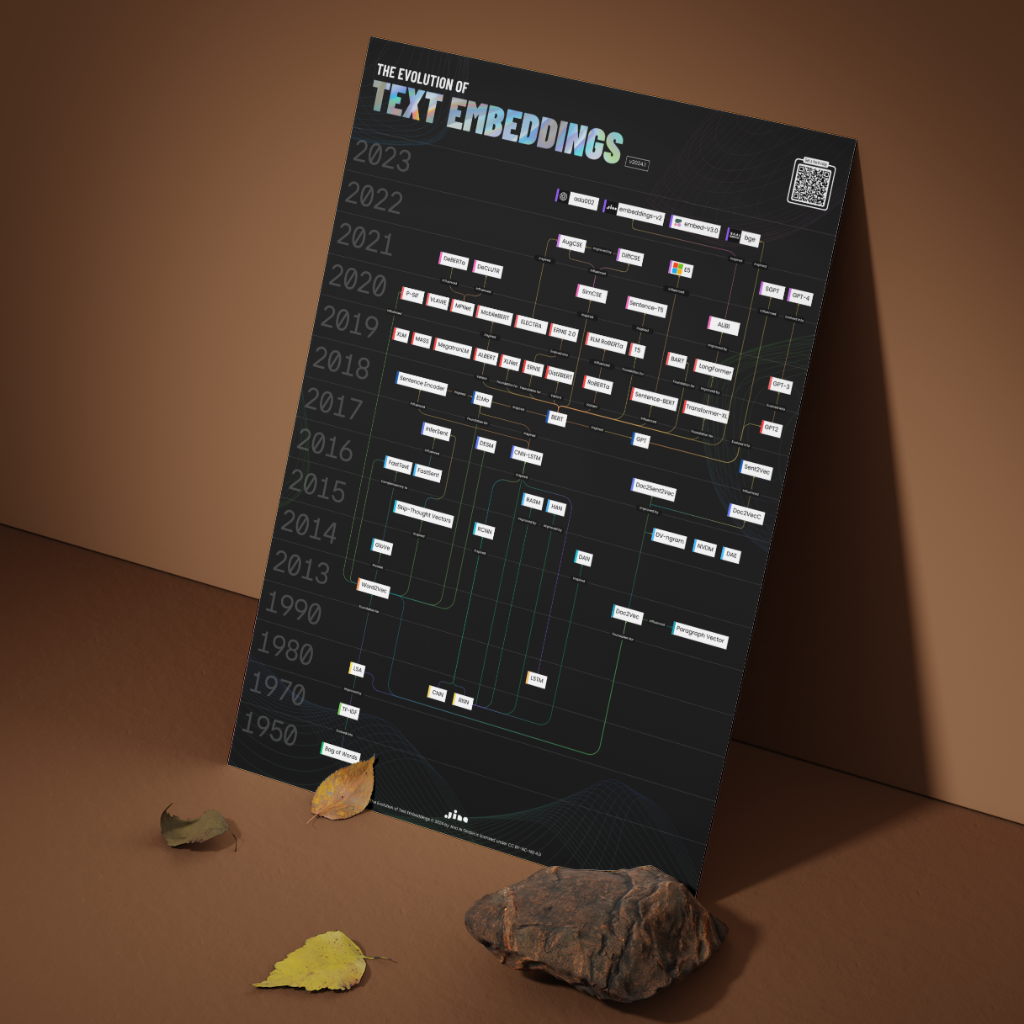
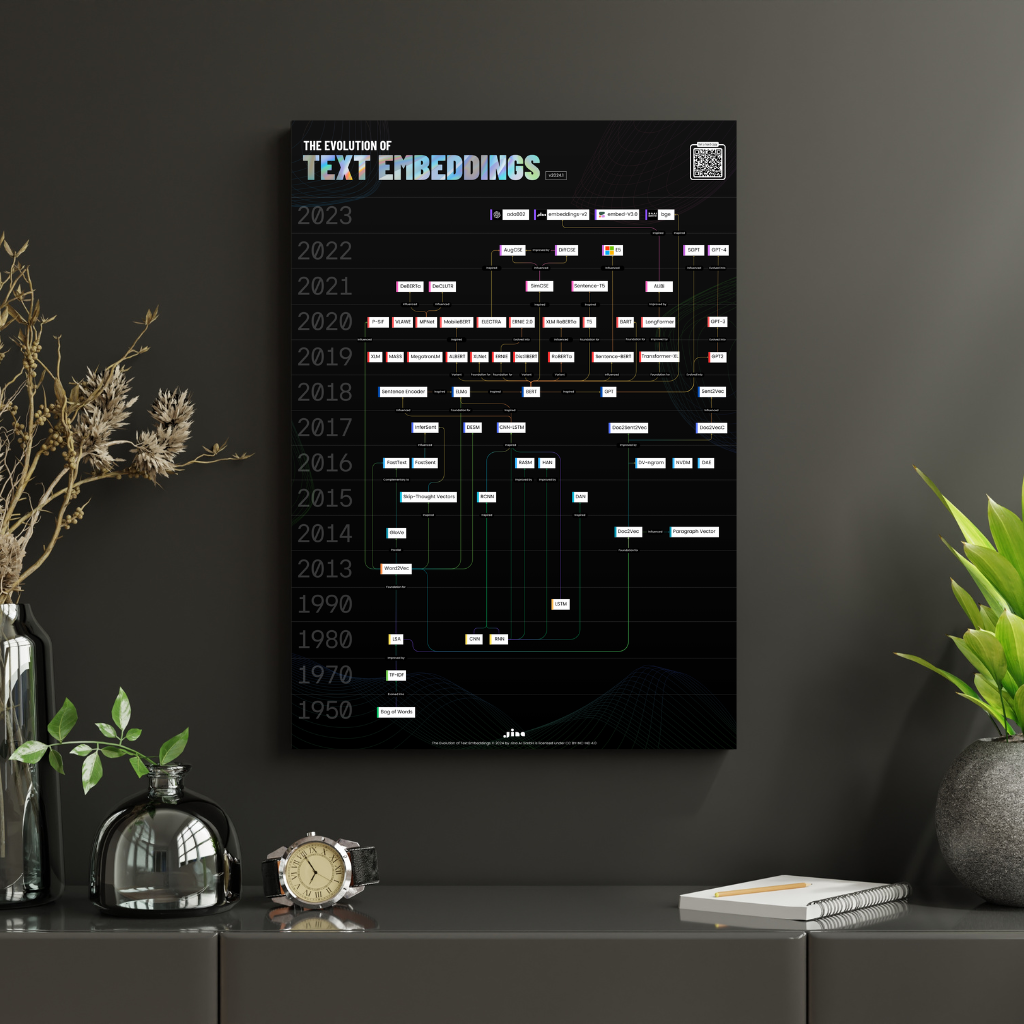
Accessible in Multiple Formats
Not ready for a physical copy? No problem. We offer a downloadable PNG or PDF version, ensuring you can access this wealth of information in the format that best suits your needs.
References at Your Fingertips
Accompanying our infographic, we provide an extensive list of references, corresponding to each milestone depicted. This curated collection allows you to delve deeper into each technology, understanding the intricacies and applications that have shaped the field of natural language processing.
TF-IDF 1972 K.S. Jones, A statistical interpretation of term specificity and its application in retrieval, J. Doc. 28 (1972) 11–21.
TF-IDF 1973 K.S. Jones, Index term weighting, Inf. Storage Retr. 9 (11) (1973) 619–633.
Bag of Words 1981 Z.S. Harris, Distributional structure, in: Papers on Syntax, Springer, 1981, pp. 3–22.
BoN-Grams 1994 W. Cavnar, W.B. Cavnar, J.M. Trenkle, N-gram-based text categorization, in: Proceedings of 3rd Annual Symposium on Document Analysis and Information Retrieval (SDAIR-94), 1994, pp. 161–175.
doc2vec 2014 Q. Le, T. Mikolov, Distributed representations of sentences and documents, in: Proceedings of the 31st International Conference on International Conference on Machine Learning (ICML) - Volume 32, ICML ’14, JMLR.org, 2014, pp. II–1188–II–1196.
DAN 2015 M. Iyyer, V. Manjunatha, J. Boyd-Graber, H. Daumé III, Deep unordered composition rivals syntactic methods for text classification, in: Proceedings of the 53rd Annual Meeting of the Association for Computational Linguistics and the 7th International Joint Conference on Natural Language Processing (Volume 1: Long Papers), Association for Computational Linguistics, Beijing, China, 2015, pp. 1681–1691.
RCNN 2015 S. Lai, L. Xu, K. Liu, J. Zhao, Recurrent convolutional neural networks for text classification, in: Proceedings of the Twenty-Ninth AAAI Conference on Artificial Intelligence, AAAI ’15, AAAI Press, 2015, pp. 2267–2273.
RNNs 2015 D. Bahdanau, K. Cho, Y. Bengio, Neural machine translation by jointly learning to align and translate, in: International Conference on Learning Representations (ICLR) 2015, 2014.
Skip-Thought 2015 R. Kiros, Y. Zhu, R.R. Salakhutdinov, R. Zemel, R. Urtasun, A. Torralba, S. Fidler, Skip-thought vectors, in: C. Cortes, N. Lawrence, D. Lee, M. Sugiyama, R. Garnett (Eds.), Advances in Neural Information Processing Systems, Vol. 28, Curran Associates, Inc., 2015, pp. 3294–3302.
DESM 2016 E. Nalisnick, B. Mitra, N. Craswell, R. Caruana, Improving document rank- ing with dual word embeddings, in: Proceedings of the 25th International Conference Companion on World Wide Web, 2016, pp. 83–84.
DV-ngram 2016 B. Li, T. Liu, X. Du, D. Zhang, Z. Zhao, Learning document embeddings by predicting n-grams for sentiment classification of long movie reviews, in: Workshop Contribution at International Conference on Learning Representations (ICLR) 2016, 2016.
FastSent 2016 F. Hill, K. Cho, A. Korhonen, Learning distributed representations of sentences from unlabelled data, in: Proceedings of the 2016 Conference of the North American Chapter of the Association for Computational Linguistics: Human Language Technologies, Association for Computational Linguistics (ACL), San Diego, California, 2016, pp. 1367–1377.
HAN 2016 Z. Yang, D. Yang, C. Dyer, X. He, A. Smola, E. Hovy, Hierarchical attention networks for document classification, in: Proceedings of the 2016 Conference of the North American Chapter of the Association for Computational Linguistics: Human Language Technologies, Association for Computational Linguistics, San Diego, California, 2016, pp. 1480–1489.
NVDM 2016 Y. Miao, L. Yu, P. Blunsom, Neural variational inference for text processing, in: Proceedings of the 33rd International Conference on International Conference on Machine Learning (ICML) - Volume 48, ICML ’16, JMLR.org, 2016, pp. 1727–1736.
Siamese CBoW 2016 T. Kenter, A. Borisov, M. de Rijke, Siamese CBOW: Optimizing word embed- dings for sentence representations, in: Proceedings of the 54th Annual Meeting of the Association for Computational Linguistics (Volume 1: Long Papers), Association for Computational Linguistics (ACL), Berlin, Germany, 2016, pp. 941–951.
CNN-LSTM 2017 Z. Gan, Y. Pu, R. Henao, C. Li, X. He, L. Carin, Learning generic sentence representations using convolutional neural networks, in: Empirical Methods in Natural Language Processing, EMNLP, 2017, pp. 2390–2400.
CNNs 2017 Y. Zhang, D. Shen, G. Wang, Z. Gan, R. Henao, L. Carin, Deconvolutional paragraph representation learning, in: I. Guyon, U.V. Luxburg, S. Bengio, H. Wallach, R. Fergus, S. Vishwanathan, R. Garnett (Eds.), Advances in Neural Information Processing Systems, Vol. 30, Curran Associates, Inc., 2017, pp. 5438–5445.
CNNs 2017 Z. Zhu, J. Hu, Context aware document embedding, 2017, arXiv:1707.01521.
Doc2VecC 2017 M. Chen, Efficient vector representation for documents through corruption, in: International Conference on Learning Representations, ICLR, 2017.
DiSan 2018 T. Shen, T. Zhou, G. Long, J. Jiang, S. Pan, C. Zhang, DiSAN: Directional self- attention network for RNN/CNN-free language understanding, in: AAAI, 2018, pp. 5446–5455.
ELMo 2018 M.E. Peters, M. Neumann, M. Iyyer, M. Gardner, C. Clark, K. Lee, L. Zettle- moyer, Deep contextualized word representations, in: Proceedings of the 2018 Conference of the North American Chapter of the Association for Computational Linguistics: Human Language Technologies, Volume 1 (Long Papers), Associa- tion for Computational Linguistics (ACL), New Orleans, Louisiana, 2018, pp. 2227–2237.
GPT-2 2018 A. Radford, J. Wu, R. Child, D. Luan, D. Amodei, I. Sutskever, Language models are unsupervised multitask learners, OpenAI Blog (2018).
ReSan 2018 T. Shen, T. Zhou, G. Long, J. Jiang, S. Wang, C. Zhang, Reinforced self-attention network: A hybrid of hard and soft attention for sequence modeling, in: Proceedings of the 27th International Joint Conference on Artificial Intelligence, IJCAI ’18, AAAI Press, 2018, pp. 4345–4352.
Sent2vec 2018 M. Pagliardini, P. Gupta, M. Jaggi, Unsupervised learning of sentence embed- dings using compositional n-gram features, in: Proceedings of North American Chapter of the Association for Computational Linguistics NAACL-HLT, 2018, pp. 528–540.
BART 2019 Lewis, Mike, et al. "Bart: Denoising sequence-to-sequence pre-training for natural language generation, translation, and comprehension." arXiv preprint arXiv:1910.13461 (2019).
BERT 2019 J. Devlin, M.-W. Chang, K. Lee, K. Toutanova, BERT: Pre-training of deep bidi- rectional transformers for language understanding, in: Proceedings of the 2019 Conference of the North American Chapter of the Association for Computational Linguistics: Human Language Technologies, Volume 1 (Long and Short Papers), Association for Computational Linguistics (ACL), Minneapolis, Minnesota, 2019, pp. 4171–4186.
DistilBERT 2019 V. Sanh, L. Debut, J. Chaumond, T. Wolf, DistilBERT, a distilled version of BERT: smaller, faster, cheaper and lighter, in: 5th Workshop on Energy Efficient Machine Learning and Cognitive Computing at NeurIPS 2019, 2019.
DocBERT 2019 A. Adhikari, A. Ram, R. Tang, J. Lin, DocBERT: BERT for document classification, 2019, ArXiv abs/1904.08398.
LASER 2019 M. Artetxe, H. Schwenk, Massively multilingual sentence embeddings for zero- shot cross-lingual transfer and beyond, Trans. Assoc. Comput. Linguist. 7 (2019) 597–610.
MASS 2019 K. Song, X. Tan, T. Qin, J. Lu, T. Liu, MASS: Masked sequence to sequence pre-training for language generation, in: K. Chaudhuri, R. Salakhutdinov (Eds.), Proceedings of the 36th International Conference on Machine Learning, ICML 2019, 9-15 June 2019, Long Beach, California, USA, in: Proceedings of Machine Learning Research, vol. 97, PMLR, 2019, pp. 5926–5936.
SBERT 2019 N. Reimers, I. Gurevych, Sentence-BERT: Sentence embeddings using siamese BERT-networks, in: Proceedings of the 2019 Conference on Empirical Methods in Natural Language Processing and the 9th International Joint Conference on Natural Language Processing (EMNLP-IJCNLP), Association for Computational Linguistics, Hong Kong, China, 2019, pp. 3982–3992.
Transformer-XL 2019 Z. Dai, Z. Yang, Y. Yang, J. Carbonell, Q. Le, R. Salakhutdinov, Transformer- XL: Attentive language models beyond a fixed-length context, in: Proceedings of the 57th Annual Meeting of the Association for Computational Linguistics, ACL, Association for Computational Linguistics, Florence, Italy, 2019, pp. 2978–2988.
VLAWE 2019 R.T. Ionescu, A. Butnaru, Vector of locally-aggregated word embeddings (VLAWE): A novel document-level representation, in: Proceedings of the 2019 Conference of the North American Chapter of the Association for Computational Linguistics: Human Language Technologies, Volume 1 (Long and Short Papers), Association for Computational Linguistics (ACL), Minneapolis, Minnesota, 2019, pp. 363–369.
XLM 2019 A. Conneau, G. Lample, Cross-lingual language model pretraining, in: H. Wallach, H. Larochelle, A. Beygelzimer, F. d’Alché Buc, E. Fox, R. Garnett (Eds.), Advances in Neural Information Processing Systems, Vol. 32, Curran Associates, Inc., 2019, pp. 7059–7069.
XLNet 2019 Z. Yang, Z. Dai, Y. Yang, J. Carbonell, R.R. Salakhutdinov, Q.V. Le, XLNet: Generalized autoregressive pretraining for language understanding, in: H. Wal- lach, H. Larochelle, A. Beygelzimer, F. d’Alché Buc, E. Fox, R. Garnett (Eds.), Advances in Neural Information Processing Systems, Vol. 32, Curran Associates, Inc., 2019, pp. 5753–5763.
ALBERT 2020 Z. Lan, M. Chen, S. Goodman, K. Gimpel, P. Sharma, R. Soricut, ALBERT: A lite BERT for self-supervised learning of language representations, in: International Conference on Learning Representations, ICLR, OpenReview.net, 2020.
ELECTRA 2020 Clark, Kevin, et al. "Electra: Pre-training text encoders as discriminators rather than generators." arXiv preprint arXiv:2003.10555 (2020).
P-SIF 2020 V. Gupta, A. Saw, P. Nokhiz, P. Netrapalli, P. Rai, P. Talukdar, P-SIF: Document embeddings using partition averaging, in: Proceedings of the AAAI Conference on Artificial Intelligence, Vol. 34, 2020, pp. 7863–7870.
P-SIF 2020 V. Gupta, A. Kumar, P. Nokhiz, H. Gupta, P. Talukdar, Improving docu- ment classification with multi-sense embeddings, in: European Conference on Artificial Intelligence (ECAI) 2020, IOS Press, 2020, pp. 2030–2037.
RoBERTa 2020 Y. Liu, M. Ott, N. Goyal, J. Du, M. Joshi, D. Chen, O. Levy, M. Lewis, L.Zettlemoyer, V. Stoyanov, RoBERTa: A robustly optimized BERT pretrainingapproach, in: Under Review as a Conference Paper at International Conference on Learning Representations (ICLR) 2020, 2020.
SpanBERT 2020 M. Joshi, D. Chen, Y. Liu, D. Weld, L. Zettlemoyer, O. Levy, SpanBERT: Improving pre-training by representing and predicting spans, Trans. Assoc. Comput. Linguist. 8 (2020).
SimCSE 2021 Tianyu Gao, Xingcheng Yao, and Danqi Chen. SimCSE: Simple contrastive learning of sentence embeddings. In Proceedings of the 2021 Conference on Empirical Methods in Natural Language Processing, pages 6894–6910, Online and Punta Cana, Dominican Republic, 2021. Association for Computational Linguistics. doi: 10.18653/v1/2021.emnlp-main.552.
AugCSE 2022 Tang, Zilu, Muhammed Yusuf Kocyigit, and Derry Wijaya. "Augcse: Contrastive sentence embedding with diverse augmentations." arXiv preprint arXiv:2210.13749 (2022).
DiffCSE 2022 Oh, Dongsuk, et al. "Don't Judge a Language Model by Its Last Layer: Contrastive Learning with Layer-Wise Attention Pooling." arXiv preprint arXiv:2209.05972 (2022).
SGPT 2022 Muennighoff, Niklas. "Sgpt: Gpt sentence embeddings for semantic search." arXiv preprint arXiv:2202.08904 (2022).
bge 2023 C-Pack: Packaged Resources To Advance General Chinese Embedding
embeddings-v2 2023 Günther, Michael, et al. "Jina Embeddings 2: 8192-Token General-Purpose Text Embeddings for Long Documents." arXiv preprint arXiv:2310.19923 (2023).